SaaS Data Analytics - Don’t Make these 4 Mistakes
For growing startups, SaaS data analytics provide insight into customer behavior. Avoid these 4 mistakes in data analysis to make clearer decisions for your product.
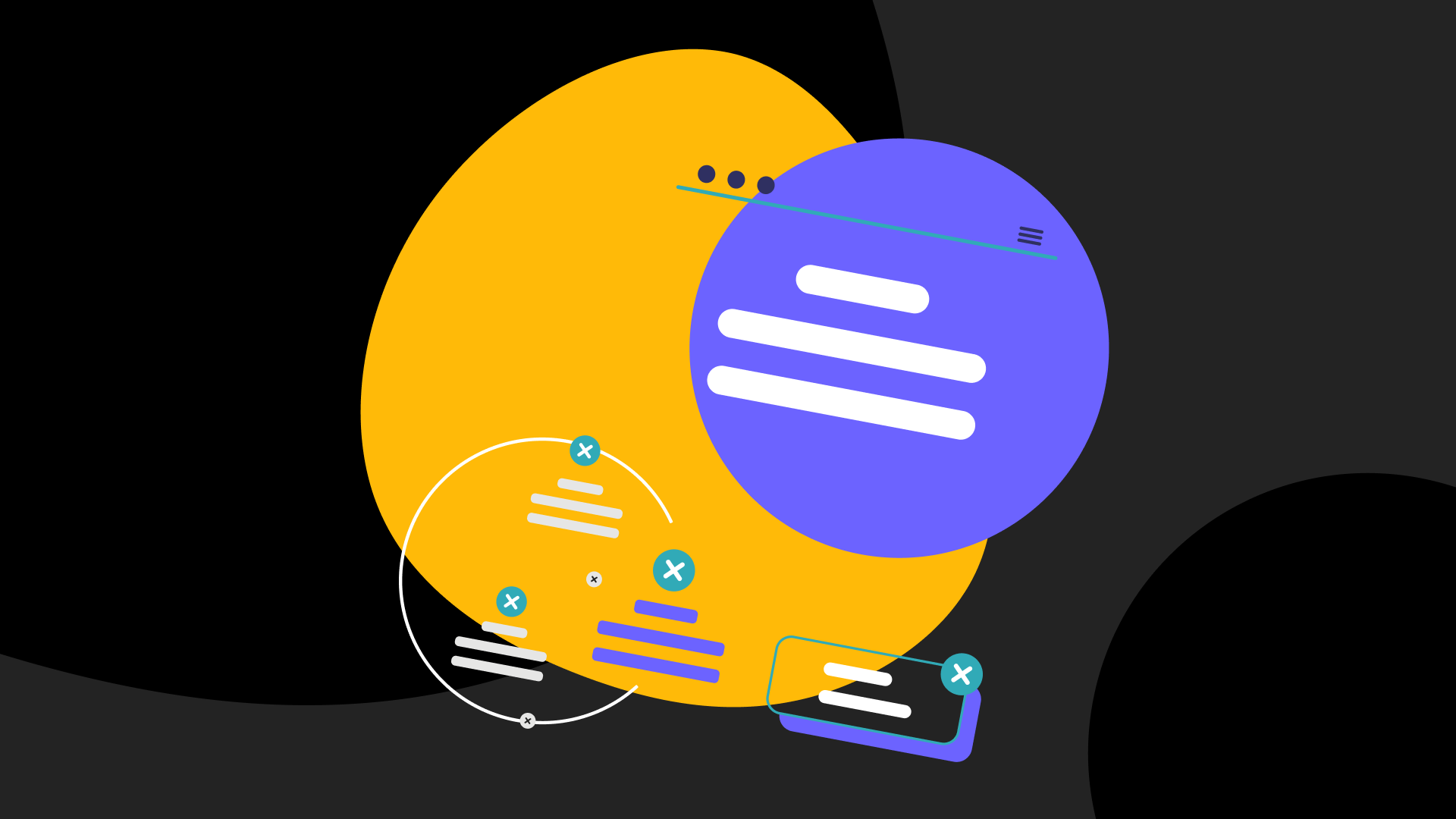
Time is of the essence in getting your SaaS data analytics set up correctly. If you don’t capture the right data now, you won’t be able to make decisions later. Since accurate retention data takes years to acquire, you can’t wait for those growth metrics to roll in to determine how your product is performing. You don’t have years to learn. You need to iterate fast to prove you have product-market fit (PMF) that’s ready to scale.
If you want to scale quickly, you must think strategically about your customers and their experience.
Prepare to adjust your mindset
Before you crack open your Saas data analytics, it’s important to take a step back and make sure you’re approaching your research with the right mindset. Learn about the four primary mistakes startups like yours make when evaluating customer data — and how to avoid them.
Common Saas data analytics mistakes
1. Drowning yourself in data without asking meaningful questions
You already know data holds the key to optimizing your product’s performance. The problem? There is so much data flowing into your system, it can be hard to mine the gold nugget insights you know must be in there…somewhere.
Raw, unsegmented data is overwhelming.
If you don’t approach it with specific questions you want to answer, you could stare at it for weeks and not parse any meaningful takeaways.
There are a number of tools out there that are meant to make your data more usable by delivering insights, including Segment.io, Mixpanel, Looker, Tableau, and Google BigQuery.
These programs aggregate and sort data into more meaningful pools, so in theory, they should provide you with useful insights. Unfortunately, that’s not the case unless you start by asking the right questions.
Identify key questions related to growth objectives
Before you open your data aggregation program, first think of the insights that would be most valuable given your current priorities or objectives.
Example key questions include:
- How many users got to the “magic moment” in your product?
- Where are most customers dropping off in the experience?
- What is the biggest bottleneck to the customers achieving value?
- What are the different types of customers we’re seeing?
- What are the key differences between our customers that succeed & customers who don’t succeed?
2. Relying on meaningless averages
SaaS analytics tools can be extremely useful, but when not used properly – they’ll really only provide you with averages. And averages are rarely useful.
Consider clothing companies… What does the average height for Americans (5”9 for men and 5”4 for women) tell you about the unique needs of real people? Absolutely nothing. Averages offer a starting point, but on their own, they won’t lead to a perfect fit on, say, a pair of jeans.
Just as a clothing company must tailor its garments for a variety of shapes and sizes – you must tailor your digital product to work for the full range of customers you serve. It’s not helpful to look at all of them through the same lens. Instead, they need to be segmented. Otherwise, you’re just averaging everyone together into the muddy middle.
How to begin segmenting users
Think about logical ways to segment your customers. While there’s no one right way to segment customers, there are some ways to start thinking about it.
For example, you could divvy them up by:
- Plan / Price point (Free, Premium)
- Context
- Job to be Done (JTBD)
- Highly engaged users versus less-engaged users
- High NPS, Low NPS
- Company Size (ie, Freelance, SMB, Enterprise)
- etc.
In some ways, you’re still trying to figure out exactly who you should be serving. Your goal when segmenting your users is to figure out who is succeeding, who is failing, what type of users you want more or less of, and how your product has to adapt for the people you want to serve.
3. Stopping at segmentation
Segmentation is a logical enough way to begin thinking about users, but if you stop there, it’s only a step better than using averages to describe customers.
Instead, you need to take your segments and divide them into cohorts. A segment, for example, might be made up of users who have created an account with you in order to be able to complete task A. Their cohort would-be users in the same boat who came in at around the same time.
Take segmentation a step further by creating cohorts
Remember that users who come into your product at different times in its development are going to have very different experiences. A user on an enterprise plan who comes in under iteration A is going to have a different set of needs and problems than a user who comes in six months later on an individual plan. In addition to being on a different plan, the product has grown and changed over the last six months.
Cohorts change the game because you’ll be able to see how changes in your product experience impact customer value and behavior over time. This is absolutely foundational for any experimentation.
4. Using lagging indicators
One of the most commonly used metrics in digital product development is a ratio that measures the relationship between a customer’s lifetime value (LTV) and the cost to acquire that customer. Ideally, it’s at or above 3:1. But there’s a big problem with acquiring that data at this early point in your product journey: it requires a lagging indicator.
Lagging indicators are often-measured metrics, but they’re not immediately helpful when trying to measure your product’s success. That’s because lagging indicators, well, lag.
Example
Lifetime value can only be determined after a customer has left your product — and if you’re doing anything right - that may take several months or even years to happen. Lagging indicators like these don’t give you immediately actionable insights.
As a relatively young startup, you don’t have the luxury of relying on long-term data. Your goal at this moment should be to quickly isolate what separates the wheat from the chaff — or the users who stay versus those who leave. Instead of using lagging indicators, it’s important to look at leading indicators or actions that, if taken, lead users to their desired outcome.
Find early leading indicators that lead users to their desired outcomes
Take Facebook. When the social media giant first got started, they quickly noticed an interesting trend based on a leading indicator. They found that users who connected with at least seven friends in their first ten days were more likely to stick with the platform and put it to regular use.
It makes sense, right? “This is where my friends are”
More immediate connections equaled more community and a greater sense of belonging, which in turn led to increased product loyalty. More photos of friends at parties or around campus, more messages sent and received, more links shared: these were all enticements that encouraged users to stick around.
This data-driven insight allowed the Facebook growth team to make smarter decisions about where to focus their efforts and how to shape their product in those critical early days. And it gave their teams a leading-indicator metric they could measure and improve upon.
Just like Facebook, you’ll want to identify your product’s “magic moment,” or the point at which customers tip from tentative explorers to devoted users. From there, you can hone in on key behaviors or milestones that predict longer-term engagement with your product. Use those to guide your product teams.
The big (data) takeaway
Now that you are pursuing growth with your product, with your customers, and with your product teams – It’s important to get your SaaS data analytics setup correctly. Growth is built on a solid foundation.
Without carefully considering your ideal audience and their different needs, you’ll wind up solving for averages and not really knowing how best to serve your customer segments. Your product’s future success depends on how quickly you learn from your current users and the feedback hidden in all the data they produce with every interaction.
To make sure you’re correctly interpreting user data to make the smartest possible decisions…
Ask yourself:
- What questions do you want to answer?
- How can you segment and cohort your users?
- How can you make sure you’re evaluating the right leading indicators?
Our discussion with Corey Haines
Your SaaS metrics are lying to you
We had the pleasure of chatting with Corey Haines, previously Head of Growth at Baremetrics and first marketing hire at Cordial. He's consulted with dozens of startups on marketing and growth including SavvyCal, Evercast, Riverside.fm, Holloway, Beamer, and Timetastic. Currently the founder of Swipefiles, which is full of cutting edge, occasionally crazy marketing ideas to grow your SaaS.
Corey shares his hot takes on SaaS metrics that don't really matter and what to focus on instead.